Why Facial Recognition?
Public security threats along with increasing security demands from users continually pressure security manufacturers to create ever improving technologies. International news constantly peppers the public consciousness with reminders of present dangers. The public wants to know – can these events ever be prevented?
For everything from safe city projects to home protection, developments are underway all over the world, with their relative obstacles. Safeguarding family members, homes, property, and vehicles are the most common applications using these emerging technologies. But the number of video surveillance cameras used in any given application, with their various networks and demanding resolutions, has increased dramatically with an equal increase in challenges. An ability to fast-track through oceans of data to find the small amount that is actually critical is now in high demand.
On the security side of video intelligence technologies, increasing customer safety has been the primary driving factor. On the business side of video intelligence technologies, increasing customer satisfaction has been the primary driving factor. Here, both service and safety are critical. Facial recognition technologies will not only address these issues, but will improve on our abilities to overcome the challenges involved. More than just speed up or enhance current methods, facial recognition will open up new avenues for security and new realms of data wherever it’s needed.
Security Industry Difficulties & Requirements
Identifying suspects during post-event investigation poses many complicated difficulties. Challenges include everything from reviewing footage from scores of cameras, comparing two face images of the same person with a 10-year age gap, and extracting the 5% (or less) of useful information from massive amounts of data, to implementing pre-event alarms to prevent incidents and managing increased video storage challenges due to increased resolutions. And likely a lot more, too.
These obstacles can prove paralyzing for standard video surveillance systems, even with up-to-date equipment. The sheer amount of information being processed bogs down system hardware. This is where more data-heavy solutions are needed, precipitating the development of deep learning and artificial intelligence products. Along with those new technologies, facial recognition is undoubtedly one of the most important right now.
Common Insufficiencies in Facial Recognition Solutions
Conventional intelligent video surveillance carries very strict requirements for a scene’s background. For human facial recognition process, two key steps are required. The first one is Feature Extraction, where the features are designed by humans and are always subjective. This method performs well in very specific environments, and subtle changes in lighting, environment, etc., sacrifice accuracy. The second step is “Classification Learning”, which uses shallow learning and analysis. Far from ideal, the results here directly restrict the breadth and depth of intelligent applications.
Deep-Learning Benefits for Intelligent Video Surveillance
Deep learning is intrinsically different from other algorithms. The way it solves the insufficiencies of traditional algorithms is encompassed in a few concepts.
First, From “Shallow” to “Deep”
The algorithmic model for deep learning has a much deeper structure than that of traditional algorithms. Sometimes, the number of layers can reach over a hundred, enabling it to process large amounts of data in complex classifications. Deep learning is very similar to the human learning process, and has a layer-by-layer feature-abstraction process. Each layer will have different “weighting,” and this weighting reflects on what was learned about the images’ “components.” The higher the layer level, the more specific the components. Simulating the human brain, an original signal in deep learning passes through layers of processing; next, it takes a partial understanding (shallow) to an overall abstraction (deep) where we can perceive the object.
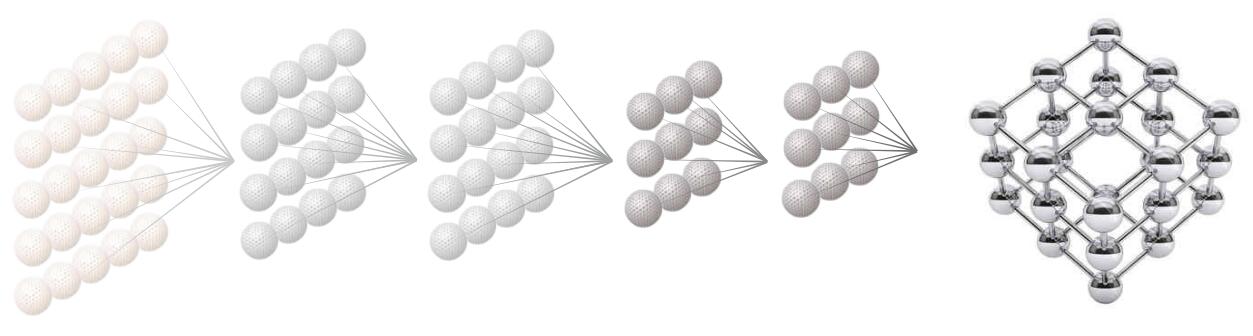
Second, From “Artificial Features” to “Feature Learning.”
Deep learning does not require manual intervention but relies on a computer to extract features by itself. This way it is able to extract as many features from the target as possible, including abstract features that are difficult or impossible to describe. The more features there are, the more accurate the recognition and classification will be. Some of the most direct benefits that deep learning algorithms can bring include achieving comparable or even better-than-human pattern recognition accuracy, strong anti-interference capabilities, and the ability to classify and recognize thousands of features.
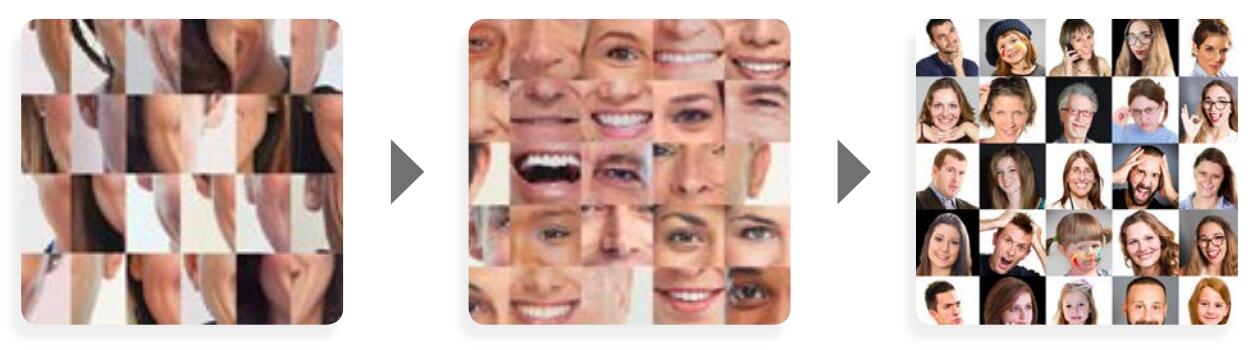
With deep learning technology, the average accuracy of facial recognition increases significantly. Hikvision has demonstrated its ability to advance ahead of the competition. Its advantages in Artificial Intelligence in security have come about by having its own excellent algorithm development team and using the most powerful GPUs in our computer platforms. The results include winning in multiple International Deep Learning algorithm competitions, such as ImageNet, PASCAL VOC, the MOT Challenge, and more.
Achieving the best results come from a combination of having the right hardware and developing algorithms that can perform the computational requirements. Hikvision has the industry's first data computing platform with best-in-class hardware, and completely independent algorithm team.
Hikvision’s Facial Recognition Solution
Hikvision’s Facial recognition Solution pushes the limits of what is possible with today’s advancements in recognition abilities. This solution detects human faces and recognises attributes create pre- and post-event face contrasting. With immense capabilities for data storage behind this solution, Hikvision’s Face Library can generate blacklists and whitelists to trigger alarms and automate entry and exit authorizations in a range of applications.
Hikvision embeds its algorithms
within the cameras and recorders, so no PC server is needed. This means less delay in transmission, decreasing the burden on back-end devices. More than just efficiency, Hikvision holds a high success rate – the face-capture rate can reach 95% accuracy, with face-comparison accuracy rate up to 98%1 . Multiple models and types are available, with a wide variety of front-end or back-end combinations to meet multitudes of scenarios and environments. And with world wide support, all human faces are supported2.
Systems integrators will see a wide range of applications becoming possible with this facial recognition solution. It can be applied in banks, luxury shops, and hotels, to recognise VIP customers and initiate specialised services. Hikvision’s solution can be applied in security scenarios, like police stations, customs, international airports, government installations, and much more, to improve security and investigation efficiency.
1Based on controlled tests; the facial recognition engine requires face images with minimum 40 pixels between the subject’s eyes (“inter-pupillary” distance), preferably 60 to 300; the maximum pan and tilt angles are 25 degrees and 15 degrees respectively for facial image capture.
2At the time of this writing, faces fitting characteristics of Asian ethnicities have the highest degree of accuracy.
Learn more about How Deep Learning Benefits the Security Industry
Other recommended articles:
Technical article – Reducing false alarms with Deep Learning
Hikvision’s Deep Learning Technology in Smart Retail Solution